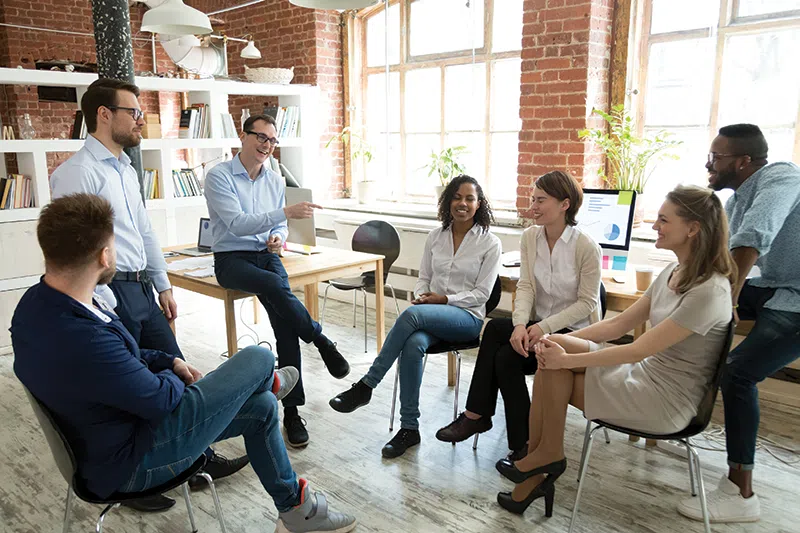
For businesses from around the world, turnover of staff is a major concern. The financial impact of replacing an outstanding worker can be high and involves recruiting costs, missed output, and the time required for educating a replacement employee. In the data-driven world of today, machine learning (ML) has grown into an effective tool for anticipating staff loss of talent, allowing businesses to take proactive measures to hold onto significant individuals. This blog discusses the value of predicting staff turnover, the various methods by which machine learning can be used to do so, and the best ways to put an efficient ML-driven plan into action.
The Importance of Predicting Employee Turnover
The productivity and profitability of a company are likely to be significantly affected by high levels of employee turnover. Employees carry significant connections, information, and abilities along with them each time they depart. In addition, it takes a lot of money and effort to recruit and educate new staff. Businesses can determine employees who are at risk and put strategies into effect that enhance job satisfaction, engagement, and retention by forecasting turnover.
It is important that you understand the factors that lead to employee turnover. Dissatisfaction with the job one has, a lack of opportunity for professional advancement, an inadequate work-life balance, and insufficient pay have many causes. However, depending on the individual in question and the organization, all of these factors might differ significantly. Here comes the possibility that a more comprehensive and data-driven method of understanding and forecasting turnover could potentially be offered by the use of machine learning.
How Machine Learning Predicts Employee Turnover
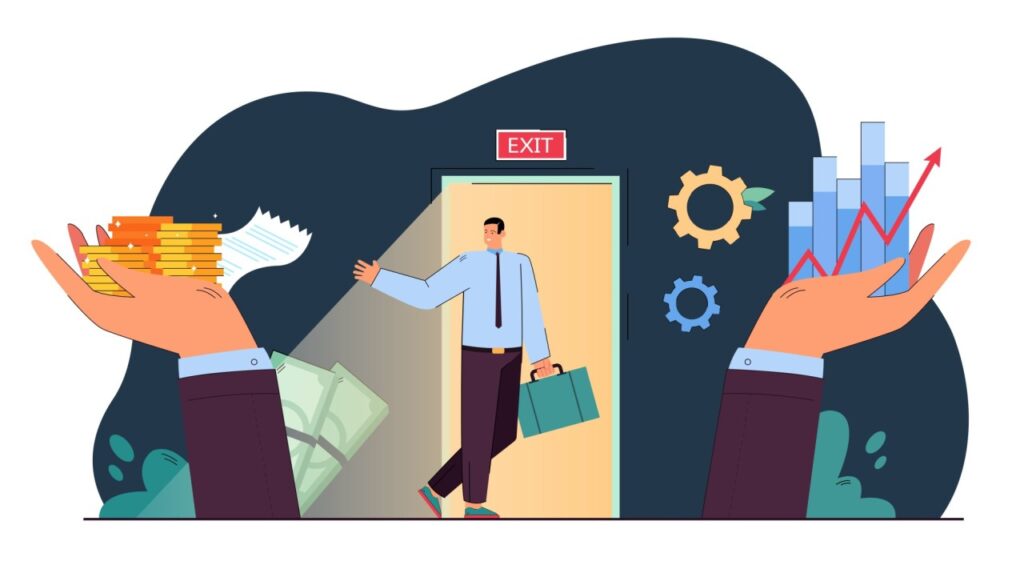
A significant amount of data can potentially be examined through models based on machine learning, which can then be used to find connections and trends that conventional methods of analysis would overlook. There are usually many steps involved in the process:
Data Collection: To be able to predict employee turnover using artificial intelligence (AI), the necessary information needs to first be gathered. The information being collected may include personal characteristics like age, gender, and educational background; work history, such as the division, tenure, and job title; measurements of performance, such as sales figures and performance evaluations; and engagement data, such as responses to surveys and involvement in business events.
Data Preprocessing: The data must be cleansed and made ready for analysis once it has been gathered. This includes addressing missing data, standardizing the data, and transforming variables with categories into numerical representations that can be processed by machine learning algorithms.
Feature Selection: When it comes to estimating turnover, all of the information is created equally. Finding the most significant factors that influence employee turnover is an important aspect of choosing the features.
Model Selection: Various techniques for machine learning, including as decision trees, random forests, support vector machines, and neural networks, can be implemented to forecast turnover. The necessary degree of prediction accuracy and the level of complexity of the data will determine which model is best. Several models are examined in order to find the best performing one.
Training and Testing: To assess the chosen machine learning model’s accuracy, it is initially trained on a particular portion of the information at hand and then evaluated on another portion of the data. Metrics like F1 score, accuracy, and recall are used to evaluate the model’s performance. Whichever workers are most likely to leave will be precisely predicted by a high-performing algorithm.
Interpretation and Action: The predicted outcomes of the algorithm may be used to identify employees who are at risk once it has been evaluated. Following that, organizations may take focused measures to address the root causes, such as providing chances for professional advancement, modifying pay, or improving work-life balance.
Best Practices for Implementing ML-Driven Turnover Prediction
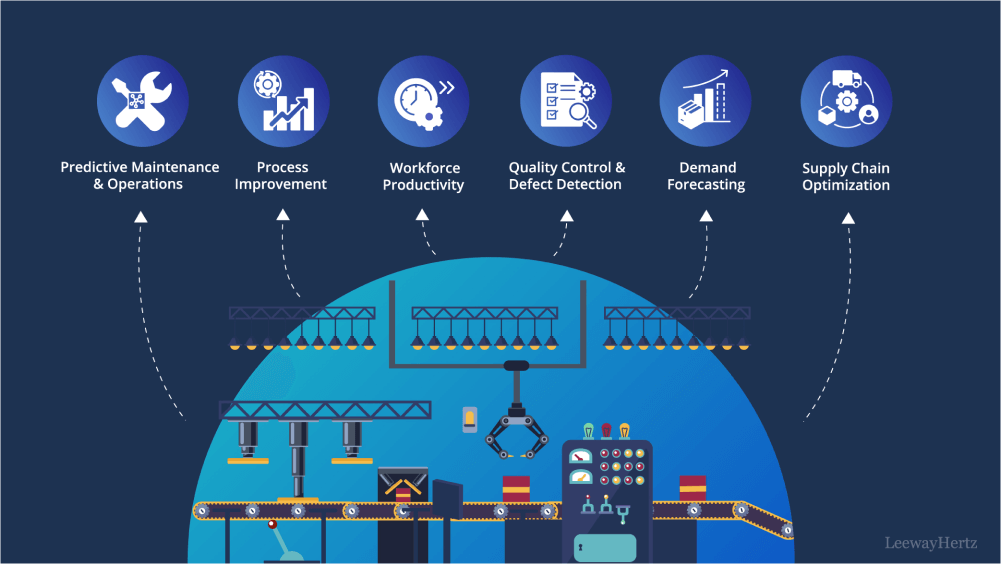
Even while machine learning has a lot of potential to forecast employee turnover, its effective application necessitates meticulous preparation and execution. Think about the following recommended practices:
Ensure Data Privacy and Security: Organizations must take precautions to manage information about staff members safely since it is important. Following through with data protection laws like GDPR and anonymizing data where it’s possible are part of this.
Involve HR and Management: It is important for researchers to collaborate with HR and management teams to construct machine learning models so that the forecasts match the goals and requirements of the company. Important insights on what factors are influencing turnover can also be obtained from these parties.
Focus on Actionable Insights: Not only does turnover prediction aim to identify at-risk workers, but it also aims to take proactive measures to keep them on board. Businesses should concentrate on using the model’s predictions to provide actionable insights and putting plans in place to deal with the risks that have been identified.
Monitor and Improve the Model: Because machine learning models are dynamic, it is important to update them regularly in order to prepare for shifts in the workforce and organization. To keep the projections accurate and relevant, constant observation and development are necessary.
Communicate Transparently: It is important to successfully communicate to staff members the objectives and advantages of the turnover forecasting model. Openness promotes trust and promises that workers are aware of the way their personal information is being used to enhance their working environment.
The Future of Employee Turnover Prediction
The use of machine learning technologies in employee turnover prediction will get increasingly complex and accurate as they develop. More individualized projections in the years to come should be expected since algorithms will account for the interests and career objectives of specific employees. Furthermore, by combining machine learning (ML) with additional technologies like sentiment analysis and natural language processing (NLP), businesses will be able to collect and examine unstructured information like employee reviews and social media interactions, which will improve the precision of turnover forecasts even more.
Last but not least, staff turnover is a challenge that machine learning can effectively address. Through the utilization of data-driven insights, companies may take proactive measures to reduce the reasons that lead to employee turnover and establish strategies for the retention of their most important staff members. Companies that integrate machine learning and predictive analytics into their business processes will be in an improved position to continue maintaining a consistent, satisfied, and effective staff as their work environment changes.